HumMUSS: Human Motion Understanding using State Space Models
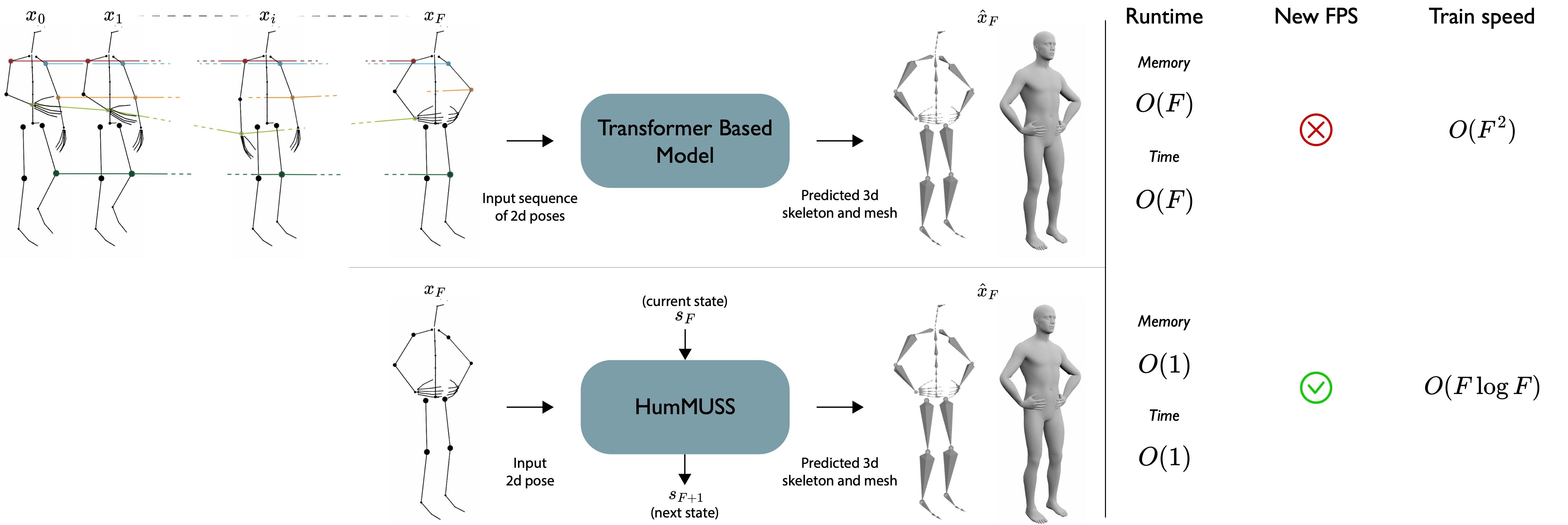
Abstract
Understanding human motion from video is crucial for applications such as pose estimation, mesh recovery, and action recognition. While state-of-the-art methods predominantly rely on Transformer-based architectures, these approaches have limitations in practical scenarios. They are notably slower when processing a continuous stream of video frames in real time and do not adapt to new frame rates. Given these challenges, we propose an attention free spatiotemporal model for human motion understanding, building upon recent advancements diagonal state space models. Our model performs comparably to its Transformer-based counterpart, but offers added benefits like adaptability to different video frame rates and faster training, with same number of parameters for longer sequences. Moreover, we demonstrate that our model can be readily adapted to real-time video scenarios, where predictions rely exclusively on the current and prior frames. In such scenarios, during inference, our model is not only several times faster than causal Transformer-based counterpart but also consistently outperforms it in terms of task accuracy.
Materials
BibTeX
@inproceedings{human-motion-understanding,
title = {HumMUSS: Human Motion Understanding using State Space Models},
booktitle = {CVPR},
author = {Arnab Mondal and Stefano Alletto and Denis Tome'},
year = {2024},
URL = {https://arxiv.org/pdf/2404.10880.pdf}
}