XR-Egopose: Egocentric 3d human pose from an hmd camera
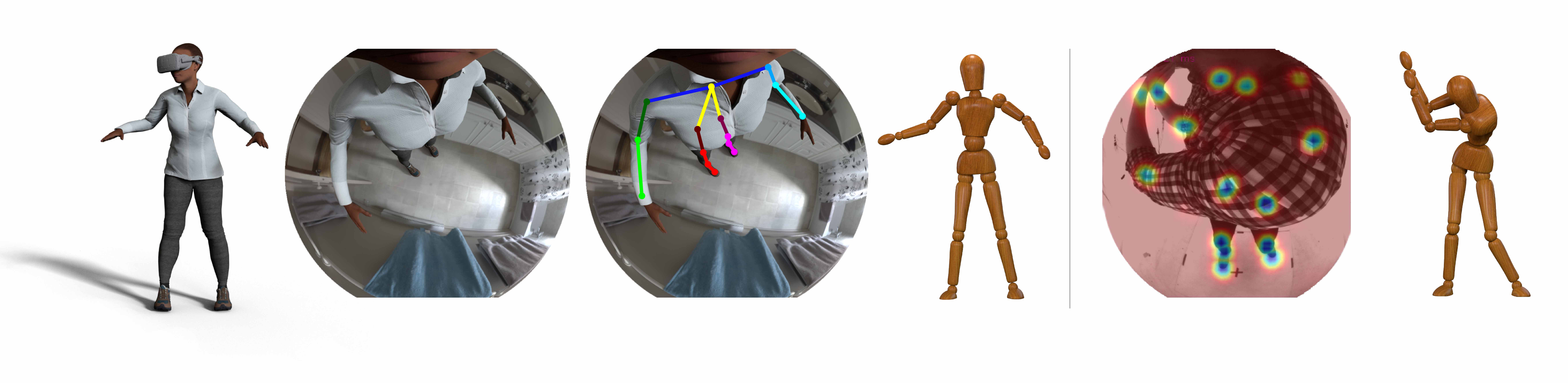
Abstract
We present a new solution to egocentric 3D body pose estimation from monocular images captured from a downward looking fish-eye camera installed on the rim of a head mounted virtual reality device. This unusual viewpoint, just 2 cm away from the user’s face, leads to images with unique visual appearance, characterized by severe self-occlusions and strong perspective distortions that result in a drastic difference in resolution between lower and upper body. Our contribution is two-fold. Firstly, we propose a new encoder-decoder architecture with a novel dual branch decoder designed specifically to account for the varying uncertainty in the 2D joint locations. Our quantitative evaluation, both on synthetic and real-world datasets, shows that our strategy leads to substantial improvements in accuracy over state of the art egocentric pose estimation approaches. Our second contribution is a new large-scale photorealistic synthetic dataset - xR-EgoPose - offering 383K frames of high quality renderings ofpeople with a diversity of skin tones, body shapes, clothing, in a variety of backgrounds and lighting conditions, performing a range of actions. Our experiments show that the high variability in our new synthetic training corpus leads to good generalization to real world footage and to state of the art results on real world datasets with ground truth. Moreover, an evaluation on the Human3.6M benchmark shows that the performance of our method is on par with top performing approaches on the more classic problem of 3D human pose from a third person viewpoint.
Dataset
This figure illustrates the challenges faced in egocentric human pose estimation: severe
self-occlusions, extreme perspective effects and lower pixel density for the lower body. The color
gradient indicates the density of image pixels for each area of the body: green is higher pixel
density, whereas red is lower density.
To faces these challenges we created a new new large-scale dataset, composed of 383K frames, focuses on realism, with augmentation of characters, environments, and lighting conditions. It departs from the only other existing monocular egocentric dataset from a headmounted fish-eye camera in its photorealistic quality , different viewpoint (since the images are rendered from a camera located on a VR HMD), and its high variability in characters, backgrounds and actions. A further advantage of this architecture is that the second branch is only needed at training time and can be removed at test time, guaranteeing the same performance and a faster execution.
Architecture
Due to the challenging task a novel architecture design is needed: a dual-branch autoencoder architecture. The first module detects 2D heatmaps of the locations of the body joints in image space using a ResNet, whereas the second module takes the 2D heatmaps as inputs and regresses the 3D coordinates of the body joints using a novel dual branch auto-encoder. One of the most important advantages of this pipeline approach is that 2D and 3D modules can be trained independently according to the available training data.
Videos
Results
Materials
BibTeX
@INPROCEEDINGS{9010983,
author={Tome, Denis and Peluse, Patrick and Agapito, Lourdes and Badino, Hernan},
booktitle={2019 IEEE/CVF International Conference on Computer Vision (ICCV)},
title={xR-EgoPose: Egocentric 3D Human Pose From an HMD Camera},
year={2019},
volume={},
number={},
pages={7727-7737},
doi={10.1109/ICCV.2019.00782}
}